The Client
The Seoul Clinical Laboratories (SCL) is South Korea’s most prominent diagnostic and reference laboratory, specialising in a wide range of medical testing and diagnostic services. Established in 1983 as the first nationwide reference lab, the group expanded into direct-to-consumer care through their Hanaro Medical Check-Up Centre, also delivering health services in other countries (China and Indonesia). With state-of-the-art facilities and a commitment to precision, SCL plays a vital role in supporting clinical decision-making and research, particularly, with focus on disease burdening the Korean population.
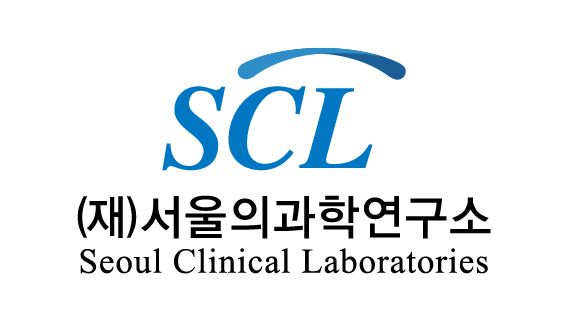
The Challenge
Metabolic syndrome (MetS) is a group of risk factors, including abdominal obesity, high blood pressure, and high blood sugar, that increase the likelihood of heart disease and diabetes. In South Korea, the prevalence is about 30% among adults, with higher rates in older populations and men. Public health initiatives focus on raising awareness and promoting healthier lifestyles to reduce these risks. Recent studies have identified genetic components that predisposes individuals to MetS, however, most of them were performed on European cohorts.
SCL wanted to identify population-specific biomarkers of MetS for the South Korean population, as genetic risk is expected to vary substantially between ethnicities. To do this, SCL recruited individuals diagnosed with MetS as well as healthy controls. The ask was to
- develop a case-control approach that works for a relatively small cohort (~800 individuals)
- that takes genome-wide interactions between genes into account
- that can be performed by SCL on hardware located in South Korea for privacy
The Solution
Commonwealth Scientific and Industrial Research Organisation (CSIRO) set up a secure bioinformatics analysis workflow within SCL’s Amazon Web Services (AWS) account. Once set up, CSIRO’s access could be revoked, enabling SCL to deposit and process their genomic samples with absolute security.
The analysis workflow utilised VariantSpark, a machine-learning approach for case-control studies with increased sensitivity in detecting associations, relative to traditional GWAS approaches, particularly for smaller datasets [1]. It was set up to run on Jupyter notebooks, making it user-friendly and easy to expand.
Though never exposed to the data directly, CSIRO supported SCL on the whole journey, from procuring an Amazon Web Services (AWS) account to using the platform with in-depth instructional documents and live demos. For the analysis, CSIRO provided sample code for data preparation and quality control as well as model selection and downstream analysis. Over the 6-month project duration, CSIRO had regular meetings with SCL to trouble shoot, adjust approaches and jointly interpreted the results.
The Outcome
CSIRO was able to provide SCL with a lead for expanding their diagnostic:
- identified MetS-assocated genes in small, well-annotated cohort: Despite the relatively small cohort of 838 samples, VariantSpark identified 159 single nucleotide variants (SNVs) significantly associated to MetS, of which the top 30 associations were further analyses downstream including gene annotation.
- identified Korean-specific markers: While the majority (87%) of the mapped genes have been previously associated with MetS, the individual SNVs itself have not been reported before. Excitingly, the VariantSpark-identified SNVs had indeed more than two-fold differences in allele frequencies in the Korean cohort compared to (non-Finnish) European populations, making them prime candidates for Korean-specific MetS biomarkers.
- identified high-confidence candidates for biomarker: one of the VariantSpark-identified SNV has been shown to be a marker for MetS in an independent South Korean cohort with 10,000 samples (12 times the cohort size).
A second cohort is currently being recruited where the cloud-based platform will allow SCL to independently conduct the analysis and validate the findings from the discovery cohort. To select the SNVs to go on the diagnostic panel, CSIRO has proposed to use its software platforms to identify interacting genes. Selecting the top interacting markers will likely be most predictive and utilises the physical space on the test most effectively.
References
- Lundberg et al. Novel Alzheimer’s disease genes and epistasis identified using machine learning GWAS platform Scientific Reports 2023